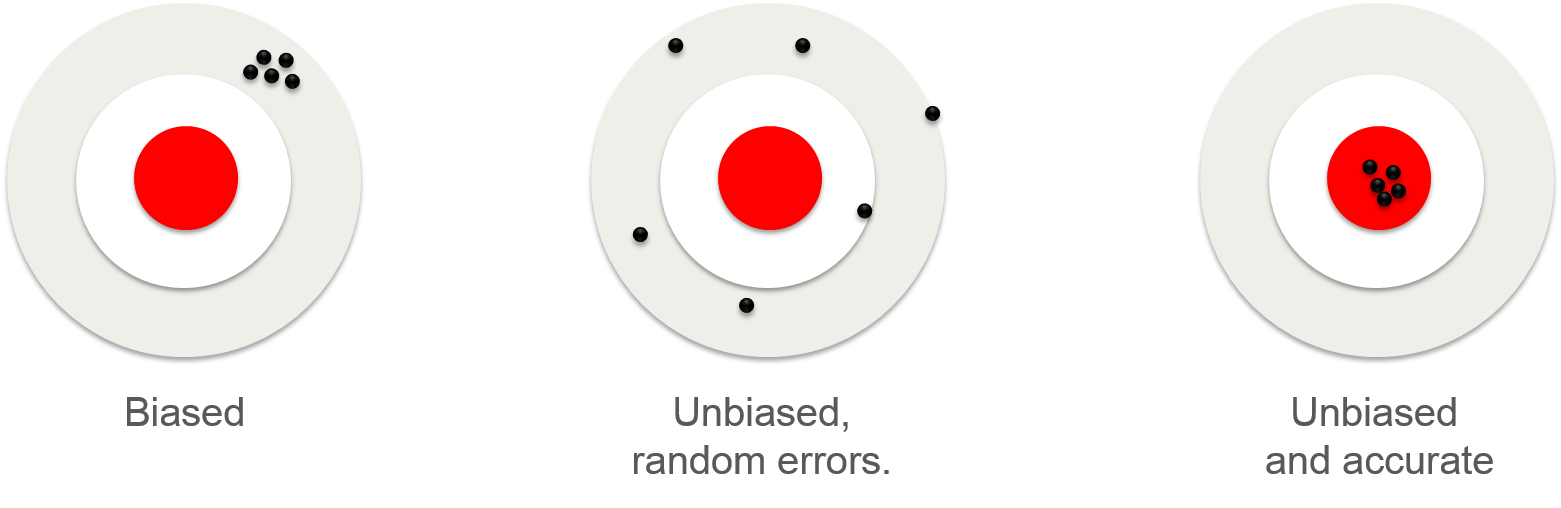
Exhibit 35.8 Inaccuracies due to systematic (bias) and random
errors.
Errors in research data can be broadly categorized into
sample and non-sample errors. Sample errors arise from the inherent inaccuracies that
occur when working with a sample of the target population. These errors can be minimized by
increasing the sample size or improving the sample design. Accuracy standards are often set
based on acceptable levels of error within a given confidence interval.
Apart from sample errors, research is also subject to non-sampling errors. These
errors occur due to various factors and can be both systematic and random in nature.
Non-sampling errors are challenging to measure and quantify accurately.
Non-sampling random errors (shown in Exhibit 35.8,
at the centre) are unpredictable errors that arise during data collection and processing.
They can result from mistakes made by interviewers, incorrect responses from respondents, or
errors in data computation during processing. These errors contribute to increased variability in
the results, but they tend to cancel out when the sample size is large.
Non-sampling systematic errors (shown in Exhibit 35.8,
on the left) accumulate across the entire sample and can lead to results that are not representative
of the population. These errors can arise from factors such as poorly worded questions that
inadvertently influence responses or issues with coverage, where certain segments of the target
population cannot be reached. Systematic errors are of greater concern as they introduce bias into
the final results. Unlike sampling inaccuracies, increasing the sample size does not reduce bias
resulting from systematic errors.
To minimize bias, the nature of the study needs to be carefully considered. For
example, in shopper trend studies, respondents should be selected in a way that neutralizes bias
arising from their proximity to shopping outlets, as shopping habits are heavily influenced by
store location.
Non-sampling error can be reduced by maintaining reliable survey frames, designing
and testing questionnaires carefully, providing thorough training to the data collection team
(interviewers, auditors), implementing monitoring and call-back procedures, and maintaining high
standards in measurement and data processing systems.